PythiaPlus
Machine learning for the study of ancient epigraphic cultures
About
The PythiaPlus research project proposes to explore and interpret the nature of the written (epigraphic) cultures of the ancient Mediterranean using Machine Learning.
Its aim is to transform our understanding of the use of epigraphic communication and the nature of cultural interference within the written and indirectly spoken languages of the ancient world. This will be achieved by revolutionising our ability to access and analyse the epigraphic data: state-of-the-art Machine Learning models will be trained to trace distinctiveness and change in the Greek and Roman epigraphic habits on an unprecedented large scale and in unparalleled detail, revealing new insights in linguistic and cultural interactions.
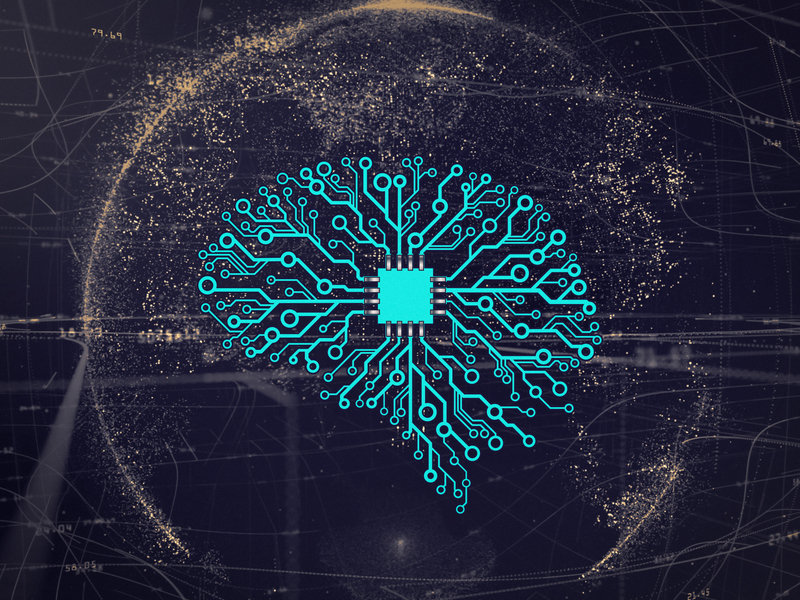
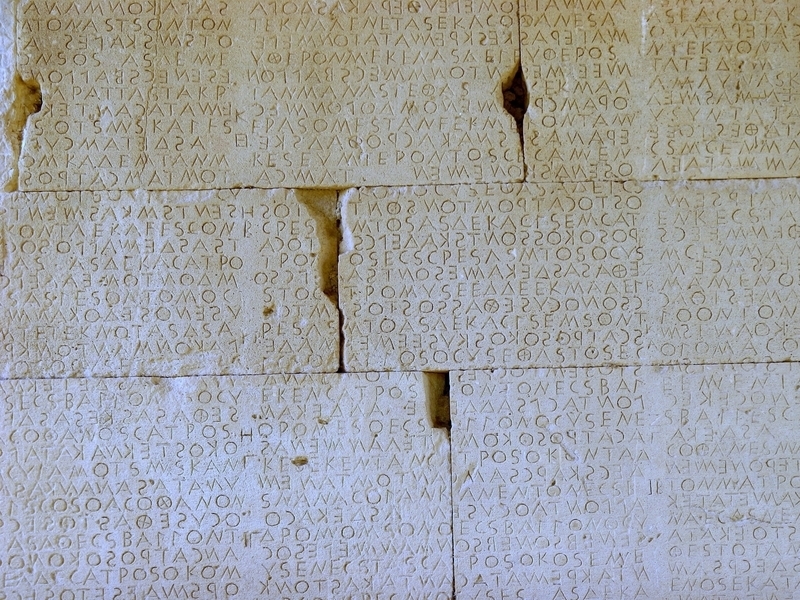
Research
Computational approaches have come to foreground or feature prominently in the research methodologies of Classical Studies and the Humanities, thus defining a unique moment and a remarkable opportunity to write an interdisciplinary history of the Graeco-Roman world in the Digital Age. Indeed, the rise of Machine Learning as a field has transformed the way data-driven research is done today, and it can meaningfully impact the way historical data is collected, analysed and interpreted.
Inscribed texts (inscriptions) in Greek and Latin are primary evidence for reconstructing the history and thought of the Ancient World due to their large number and variety in content, forming a crucial repository of textual material culture. Machine Learning models could now reveal patterns in this data which historians were previously unable to identify in such detail and on such scale: PythiaPlus will enable the first "big data" study of epigraphic cultures over circa 1,500 years of ancient Mediterranean history using Machine Learning models.
The project is articulated into 3 research work packages:
- Dataset building: gather, sample and prepare the epigraphic data to be used by Machine Learning models, rendering machine actionable the largest digital corpora of Greek and Latin inscriptions.
- Model training: train models on the data, evaluating their performance and tuning the model’s parameters to improve statistical performance and explainability. The tasks tackled will range from textual restoration and topic modelling, to the study of epigraphic metadata concerning the time and place of writing of Greek and Roman inscriptions.
- Result interpretation: interpret the new patterns discovered by the models in light of scholarly approaches to distinctive and distinct epigraphic habits.
PythiaPlus’ expected results are:
- To deliver educational and research tools, the open-sourced machine learning models and the two standardised epigraphic datasets, to track textual connections and make the machine actionable data more accessible for future research.
- To advance the state of the art in the contextualisation of written (epigraphic) evidence and the reconstruction of ancient epigraphic habits, also projecting the patterns discovered by the machine learning models onto evidence whose attribution is uncertain, as in the case of newly discovered, looted or irreparably damaged documents.
- To pioneer a new approach to the analysis of textual material cultures using machine learning, applying the latest technological advances to the study of ancient artefacts and documents and drawing from both historical and digital methodologies.
Outputs
The first output of the PythiaPlus project is Ithaca, the first deep neural network for restoring, dating and placing ancient Greek inscriptions. This research is the result of a collaborative effort between Ca' Foscari University of Venice, DeepMind, the University of Oxford and the University of Athens AUEB. The model was designed with collaboration in mind and is best used in conjunction with researchers where historical knowledge combines with Ithaca’s assistive input. While Ithaca alone achieves 62% accuracy when restoring damaged texts, when historians use it their performance leaps from 25% to 72%. Ithaca can also attribute inscriptions to their original location with 71% accuracy and date them with less than 30 years from ground-truth ranges. Machine Learning models like Ithaca can unlock the cooperative potential between AI and the humanities, transformationally impacting the way we study and write about ancient Greek history.
The article was published on the cover issue of Nature (vol. 603, no. 7900, 10 March 2022) and received media coverage worldwide (The Times, The Guardian, New Scientist, La Repubblica, Kathimerini, DeepMind, Ca’ Foscari News, the Greek Ministry of Culture).
To make the research widely available to researchers, educators, museum staff and others, the team partnered with Google Cloud and Google Arts & Culture to launch a free interactive version of Ithaca, and also open sourced the code, the pretrained model, and an interactive Collaboratory notebook.
- Read the article (open access)
- Watch the promotional video (curated by Nature)
- Use Ithaca’s interface for your own research
- Check out Ithaca’s code
- Cite the article: Assael, Y.,* Sommerschield, T.,* Shillingford, B., Bordbar, M., Pavlopoulos, J., Chatzipanagiotou, M., Androutsopoulos, I., Prag, J., de Freitas, N. “Restoring and attributing ancient texts with deep neural networks.” In Nature, 603(7900). Cover issue of 10 March 2022. https://doi.org/10.1038/s41586-022-04448-z
Secondments
- DeepMind is one of the world's leading organisations in artificial intelligence research. Its Research Teams use Google’s computing infrastructure to establish an unparalleled track record of AI breakthroughs resulting in hundreds of peer-reviewed papers.
- The Department of Informatics of the Athens University of Economics and Business (AUEB) is one of the largest Computer Science departments in Greece. I will be hosted by the Information Processing Lab and I will be joining the Natural Language Processing Group.
- The Digital Curation Unit (DCU) is part of the Information Management Systems Institute (IMSI) under the “Athena” Research Centre. It conducts research, develops technologies and apps, and acts as a national focus point in the field of digital curation (such as the APOLLONIS infrastructure for Digital Humanities).