AI MODELS
Advancing the Historical Epistemology of Artificial Intelligence
Project
The ERC project AIMODELS (full title: ‘The Culture of Algorithmic Models: Advancing the Historical Epistemology of Artificial Intelligence’) is hosted at the Department of Philosophy and Cultural Heritage of Ca’ Foscari University in Venice (2024-2028) and investigates the combined socio-technical history of contemporary AI models and models of intelligence.
This project intersects the current debates on AI from a new angle. The study of the automation of so-called human ‘intelligence’ is not separated from the formalisation and measurement of labour, language, knowledge, and social relations that often predate automation. The techniques of the division of labour already inspired the mechanisation of mental labour in Charles Babbage’s early design of Calculating Engines (Braverman 1974, Daston 1994) during the industrial age. In a similar way, psychophysics and later psychometrics, attempted a first metrology of intellectual labour (Schaffer 1999, Schmidgen 2002) to aid automation. The rise of AI cannot be separated from the government of social hierarchies of ‘hand and head’ and the discrimination of race, gender, and class, as Stephen Jay Gould (1981) already registered in the controversial practices of the IQ test and psychometrics.
The modelling of intelligence has to be problematized within the long history of modern rationality, comprehending the philosophy of mind, the codification of linguistics and semiotics, the normative power of statistics, psychometrics, and neuroscience, the rise of information technologies and computer science. Eventually this evolution contributed to a planetary regime of data monopolies based on the knowledge extractivism of cultural heritage and mass communication: AI emerged, in different ways, as a macro-paradigm that consolidated such a heterogenous history.
The study of AI should go back, at this point, to investigate the economic and social roots of modern rationality, which has never been simply a theoretical affair (Hessen 1931). In fact, the history of rationality has been always related to the material tools, machines, and practices that made it possible. Descartes’ method, to bring a paradigmatic example, was mechanistic before being mathematical: the roots of his philosophy can be traced back to machine building as Henryk Grossmann (1946) noted.
Before being considered as an innovative artefact, the idea of machine intelligence has to be considered an extension and implementation of modern mechanical thinking, which, as feminist epistemologists note, was in itself also a vision of social governance. Hilary Rose (1976), Sandra Harding (1986), Evelyn Fox Keller (1985), and Silvia Federici (2004), among others, have explained the rise of modern rationality and mechanical thinking (to which AI also belongs) in relation to the rule of women’s bodies and the transformation of the collective body into a docile and productive machine.
In short, against the anthropomorphism of AI (and myths of ‘superintelligence’), the project has the mission of historicising AI by focusing on a specific ‘epistemic object’ that is the theories and practices of modelling in between natural and social science, linguistics and computer science, statistics and digital humanities. In this way, it sees the emergence of the paradigm of AI not as a recent phenomenon but as related, as said earlier, to practices of formalisation and measurement of labour, language, knowledge, and nature at large.
Ultimately, from the vantage point of corporate AI and its outcomes (DALL-E, Midjourney, ChatGPT, etc.), a large-scale morphological transformation of culture and education has to be considered, which is comparable to the scale of the transformation of mass culture in the 20th century. After we shaped the way they operate, machines, media, and milieux affect the way we think. They also affect the way labour and society are organised, opening up new pathways to the historical process of divorcing producers from the means of production.
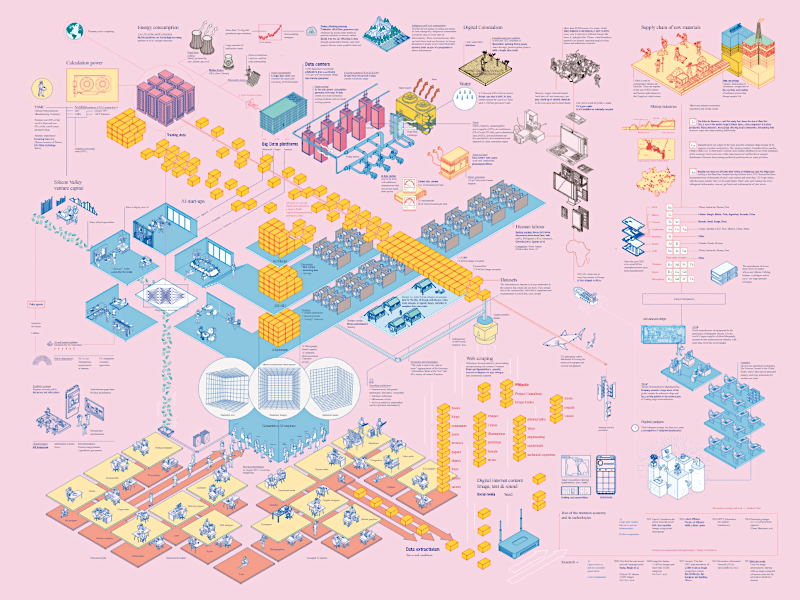
The project pursues three main objectives:
- Writing a new history of AI as a history of the definitions and metrics of intelligence that would highlight the key role of translation practices as much as technical models (in particular algorithmic models) in the evolution of statistics, computer science, digital humanities, and the current AI models of AI. This part of the project focuses on the historiographical gaps in the history of the paradigms of connectionism, artificial neural networks, and deep learning, especially since the time of the invention of the first operative neural network, the Perceptron, by the US psychologist Frank Rosenblatt.
- Building a comparative epistemology of AI that engages with the psychology of learning and development, the historical epistemology of science and technology, and the role of mental models, technical models, and models of the mind in the work of scientists, computer scientists, psychologists and educators. In this regard, the project engages with the genetic epistemology of the psychologist Jean Piaget (1968) and historian of science Peter Damerow (1996). Damerow in particular gave a key contribution to interpreting the evolution of technology and science, as a continuous cycle between the internalisation of technical models and externalisation of mental models. On the other hand, as Castelle and Reigeluth (2021) stress, human and machine learning are already co-evolving and therefore a ‘social theory of machine learning’ will become, soon, unavoidable.
- Evaluating the impact of the current large multi-purpose AI models (DALL-E, ChatGPT, etc.) on knowledge production, creativity, education, translation, and cultural heritage at large. The recent application of such models in the most diverse tasks is already impacting visibly and invisibly the everyday life of millions of people (any search performed on Google Search, for example, has been processed by the BERT model since 2019). However, what is often overlooked is the source that these AI models often parasite, which can be identified in vast unregulated repositories of cultural heritage and mass communication. Under this respect, the project argues that AI is not so much about the imitation of individual intelligence in solving problems, rather of collective intelligence and cultural heritage’s contribution in such problem solving.
Through consolidating a theory of automation based on modelling, the research will help situate AI in the horizon of the technosphere and in the long history of cultural techniques and knowledge systems, yet the ultimate purpose of the project is more specific. The research methodology positions AI as part of the evolution of modern technoscience (Pasquinelli 2023) and extends the methods of the historical epistemology of science and technology (Omodeo 2019, Renn 2020; Ienna, 2023) to AI studies. Born at the crossroads of techniques and disciplines of the past century, AI has become a central paradigm also in the disciplines of the Anthropocene and its scale of system thinking (Rispoli 2020). The reference to the Anthropocene is not gratuitous, as the problematics of modelling are central to climate science. We can perceive the planet as a system, measure climate change and make political decisions only thanks to the mediation of mathematical models.
As Paul Edwards (2010, xiii) wrote: “Today, no collection of signals or observations — even from satellites, which can “see” the whole planet — becomes global in time and space without first passing through a series of data models. [T]he models we use to project the future of climate are not pure theories, ungrounded in observation. Instead, they are filled with data — data that bind the models to measurable realities. [...] Everything we know about the world’s climate — past, present, and future — we know through models.” This observation can be extended also to the epistemology of AI.
Preliminary research themes
AI history
- The invention of algorithmic models
History of connectionism and deep learning - The history of the idea of intelligence
Neuronormativity from the school to the society - The mathematics of labour, nature, and knowledge
Metrics as the rationale of automation, computation, and extractivism
AI epistemology
- Language between formalisation and automation
Semiotics as the history of information technologies and AI - Language as the model of reason and unreason
Semiotics as the history of cognitive sciences and AI - The model of model
Comparative epistemology of paradigms, rules, and models in art, history, and science
AI culture
- What is a pattern?
Evolution of symbolic forms in art, culture, and computation - AI and poetry
Literary avant-garde and modernism studies after generative AI - Cultural heritage in (machine) translation
Politics of language between bordering and extinction
Preliminary bibliography
- Braverman, Harry (1974) “Labor and Monopoly Capital: The Degradation of Work in the Twentieth Century”, New York: Monthly Review Press.
- Castelle, Michael, and Tyler Reigeluth (2020) “What Type of Learning is Machine Learning?” in: Michael Castelle and Jonathan Roberge (eds) “The Cultural Life of Machine Learning: An Incursion Into Critical AI Studies”. Berlin: Springer.
- Damerow, Peter (1996) “Abstraction and Representation: Essays on the Cultural Evolution of Thinking”. Dordrecht: Kluwer.
- Daston, Lorraine (1994) “Enlightenment Calculations.” “Critical Inquiry” 21(1), 182–202.
- Edwards, Paul (2010) “A Vast Machine: Computer Models, Climate Data, and the Politics of Global Warming”. MIT Press.
- Federici, Silvia (2004) “Caliban and the Witch: Women, the Body and Primitive Accumulation”. New York: Autonomedia.
- Gould, Stephen Jay (1981) “The Mismeasure of Man”. New York: Norton & Company.
- Grossmann, Henryk (1946) "Descartes and the Social Origins of the Mechanistic Concept of the World" in: Gideon Freudenthal and Peter McLaughlin, eds. “The Social and Economic Roots of the Scientific Revolution: Texts by Boris Hessen and Henryk Grossmann”, Berlin: Springer, 2009.
- Keller, Evelyn Fox (1985) “Reflections on Gender and Science”. New Haven: Yale University Press.
- Harding, Sandra (1986) “The Science Question in Feminism”. Cornell University Press.
- Hessen, Boris (1931) "The Social and Economic Roots of Newton’s Principia" in: Gideon Freudenthal and Peter McLaughlin, eds. “The Social and Economic Roots of the Scientific Revolution”, cit.
- Ienna, Gerardo (2023) “Genesi e sviluppo dell'épistémologie historique: fra epistemologia, storia e politica”. Lecce: Pensa Multimedia.
- Omodeo, Pietro Daniel (2019) “Political Epistemology: The Problem of Ideology in Science Studies”, Berlin: Springer.
- Pasquinelli, Matteo (2023) “The Eye of the Master: A Social History of Artificial Intelligence”. London: Verso.
- Piaget, Jean (1968) “Genetic Epistemology”. New York: Columbia University Press.
- Renn, Jürgen (2020) “The Evolution of Knowledge: Rethinking Science for the Anthropocene”. Princeton University Press.
- Rispoli, Giulia (2020) "Genealogies of Earth System thinking", “Nature Reviews Earth & Environment” 1, 4-5.
- Rose, Hilary, and Steven Rose (eds) (1976) “The Radicalisation of Science”. London: Macmillan.
- Rosenblatt, Frank (1962) “Principles of Neurodynamics: Perceptrons and the Theory of Brain Mechanisms”, Spartan Books, Washington.
- Schmidgen, Henning (2002) "Of frogs and men: the origins of psychophysiological time experiments, 1850-1865", “Endeavour” 26(4), 142-8.
- Schaffer, Simon (1999) “OK Computer”, in: Michael Hagner (ed) ”Ecce Cortex: Beitraege zur Geschichte des modernen Gehirns", Göttingen: Wallstein Verlag, 254-85.